When faced with a complex water resources challenge, a typical reflex is to start with developing a numerical model. I have found this counterproductive.
Models certainly have their utility, but not in a water program’s conceptualizing phase. At this stage, efforts are best focused on identifying key water resources issues and on better understanding their extent, severity, and causal structures and the associated drivers, impacts, and risks.
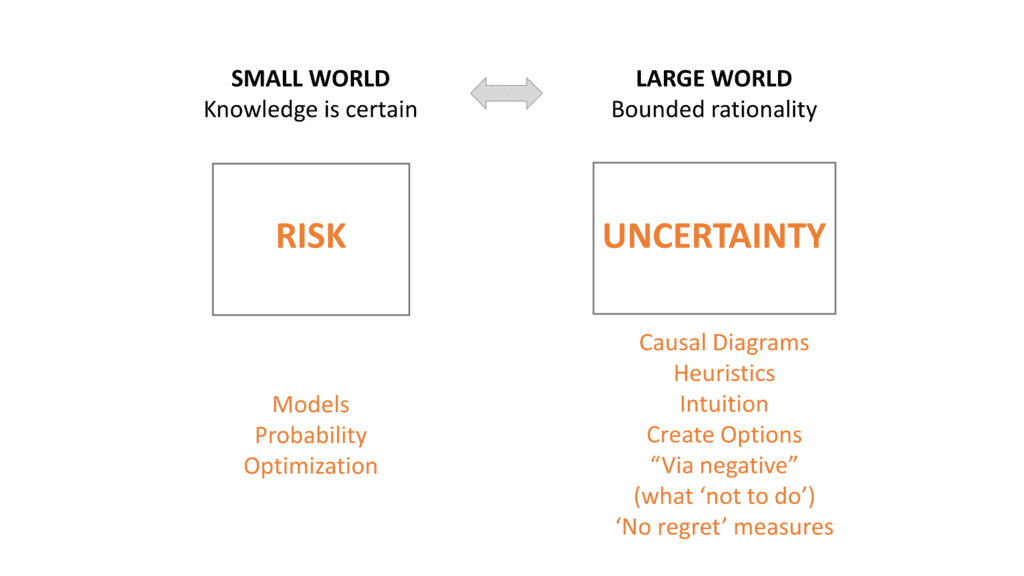
I was reminded of this observation when discussions on the validity of a hydrological model dominated an expert meeting and (almost) discredited a wide array of other project outputs. Among these other outputs was a ‘program of measures’ that would address key water resources issues.
In this specific project, model development had been hampered by a severe lack of field data. The modelers ‘persisted’ but came to rely almost exclusively on proxy indicators and large-scale and international data sets (with, inevitably, a comparatively low resolution). To make matters worse, a physically-based distributed hydrological model was selected that required lots of data.
The proposition that the hydrologic parameters—such as surface runoff and groundwater recharge—can be derived from terrain properties, without proper calibration with field observations, has unfortunately proved untrue. In this specific model, field observations were so scarce that large sections of the model were, in fact, not calibrated. Worse, the few scattered field observations that were available contradicted the model results but were subsequently ignored.
Implications
So, what did this particular modeling exercise achieve? A summary:
- A long delay in project implementation. In effect, model development monopolized the water resources analysis. All other project activities—that were supposed to use the model results as input—were stalled for over 9 months.
- Many local (but nevertheless important) water challenges were ignored because the model was based on low-resolution data that generalized the analysis. One can argue that the model “proved what we already knew”.
- A stunted conceptualization of the key water management issues. With most intellectual energy focused on model development—concerned only with climatological and hydrological parameters—non-biophysical elements in the analysis (such as legal and regulatory environment, law enforcement, stakeholder participation and motivation, or socio-economic context) received inadequate attention and were ignored in practice. Hence the conceptual analysis was inevitably incomplete.
- Reduced solution space. By not recognizing and addressing all fundamental constraining factors (see above), the proposed program of measures is at risk to be ineffective while some options (the non-biophysical ones) were not systematically explored. It affects the credibility as well as the effectiveness of the proposed program.
- Confusion. Inevitably, the partially incorrect and incomplete conceptual analysis did not create a consensus and communality of views among key stakeholders—which is a prerequisite for public action—on how to address the respective water resources issues.
- Mistrust in the resulting program. Because some key stakeholders implicitly rejected the model setup and results, the credibility of the proposed program of measures was compromised.
- Less support for the implementation of the program. A direct and obvious consequence of the loss of credibility in the analysis (see above).
The above only includes negatives. That was not my intention, and I certainly see the value of models in a well defined context. A positive outcome of the exercise is the widely accepted notion that better water data are needed.
Nevertheless, the modeling exercise was over-ambitious and poorly timed, and adversely impacted on the development of the water resources management plans. Developing a comprehensive model that ‘integrates everything’ was simply not the right thing to do at the conceptualization phase of developing water resources management plans. It’s an important ‘lessons learned’.
Make models simple in situations of uncertainty (bounded rationality)
Causal diagrams in combination with a facilitated dialog process
So, what is an alternative approach?
The first step is to make a sound conceptual analysis of the critical issues. Given the nature of water resources—which are related to so many aspects of the economy and environment—this conceptual analysis is necessarily ‘holistic’. This approach acknowledges that many solutions to ‘water problems’ are outside the water domain and related to, for instance, rural development or agricultural trade policies. For purposes of clarity, the conceptual analysis is best presented as causal diagrams. It facilitates discussions with a broad group of stakeholders to solicit their views and input.
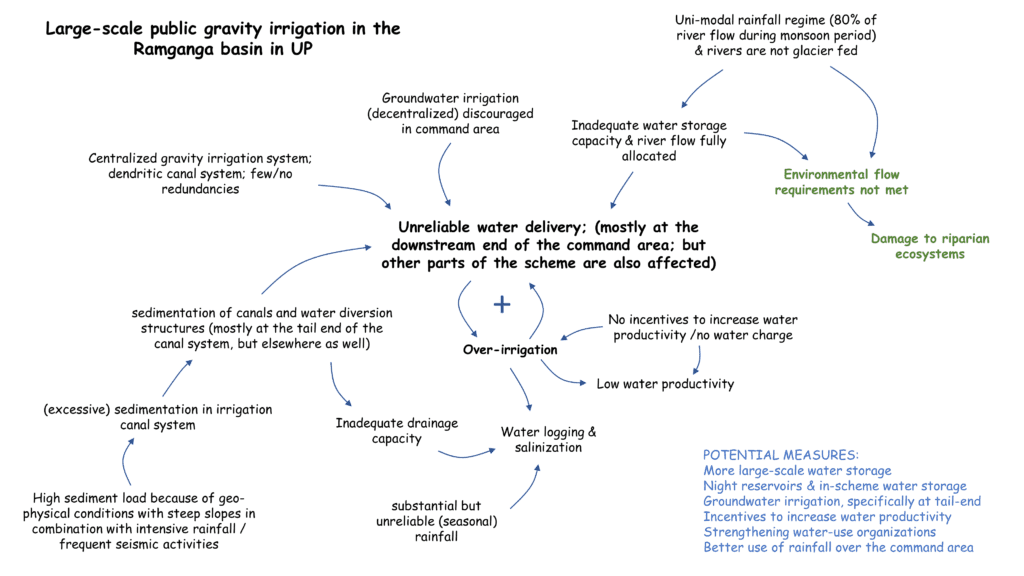
In my experience, the most effective approach to conduct this conceptual analysis and develop the causal diagrams is through a series of interviews with key stakeholders and resource persons. Once a draft of the causal diagrams has been prepared, they can be reviewed through a facilitated dialogue process (workshops) that validates and enriches the conceptual analysis and creates a shared understanding. The latter is a key requirement for public action.
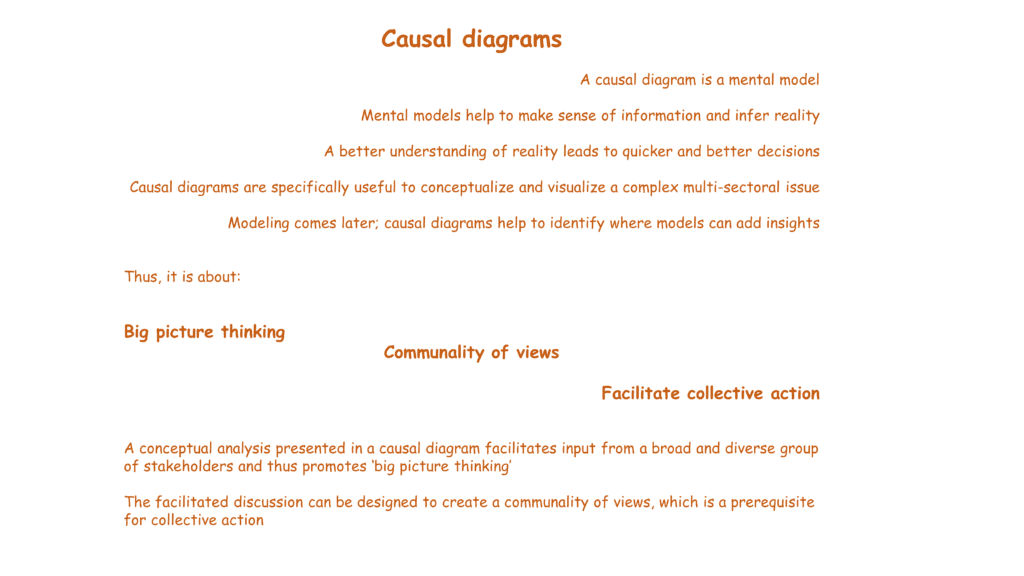
Only at this point—when there is an adequate level of consensus on the conceptual analysis—should we start thinking on where ‘numbers are needed’, and on how to get them.
In many cases, this will not require a model. If it does, this often concerns a targeted model for a bounded issue that is relatively small in geographic scope and that does not require integration with other models. It goes without saying that model development, if any, should be contingent on data availability.
The above approach builds on the collective knowledge of a large group of stakeholders and is less resource intensive (in terms of data, time, and experts), while in many cases leading to a better analysis that also includes the non-biophysical parameters. Further, the facilitated dialog process builds a communality of views and credibility with key stakeholders that will support the actual implementation of the proposed measures.
Related to the above, the approach may contribute to increasing the credibility of government institutions, which is quite necessary since low trust in government in some places is among the reasons why water resources management is so difficult at times.
An example of intuitive decision making
In the early 90s I worked as a junior design engineer on the storm-surge barrier for Rotterdam Harbor. The design followed a probabilistic approach that aimed to optimize all structural elements—from the smallest to the largest—to have uniform strength and reliability. And, of course, to control costs. It was a highly complex process.
When the design was completed, a decision was taken to increase the height of the barrier by a full meter to accommodate the potential impacts of climate change. Note that this was in the early 1990s.
The arbitrary value of 1 m contrasted with the highly detailed calculations—with cm precision—that had determined the height of the barrier. It almost felt like a random number and was certainly not based on sophisticated computations and modeling.
But, given what we now know about climate change, it was absolutely the right thing to do. I also think they got the number—a 1 m increase—quite right. It provides an example of very effective intuitive decision making that led to a robust decision. Given the intended lifespan of the barrier, the minor potential losses of not finding the ‘optimal’ decision have long since been forgotten.
What is a good decision under uncertainty? A robust one or an optimal one?
Optimization is a fiction in an uncertain and dynamic environment (Gerd Gigerenzer)
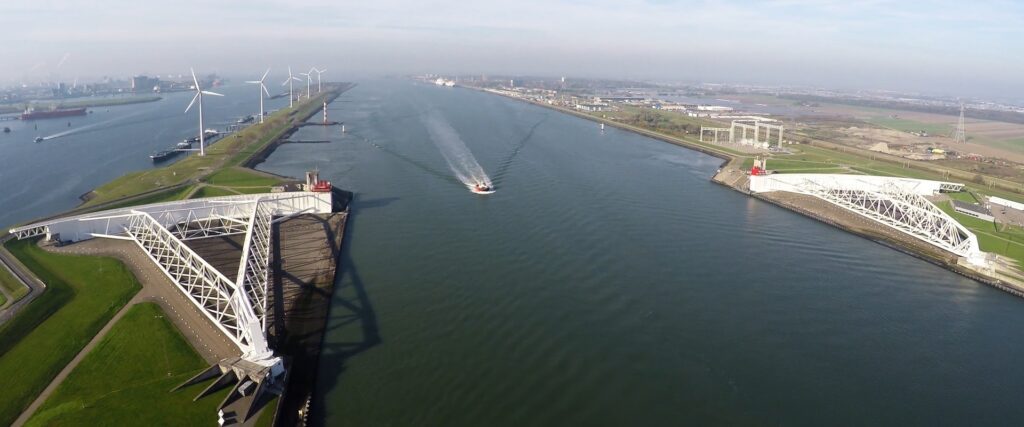
Final observation
A final observation: “the more complex a problem, the less need for a model”. Models perform excellently under bounded and well-defined conditions but have less value when trying to understand a highly complex, multi-faceted, dynamic, and integrated water resources problem.